Part 1: The Growing Challenge of Knowledge Loss in Industrial Companies Aging Workforce and Its Consequences
As the U.S. government ramps up its efforts to bring industrial manufacturing back to American soil and reestablish the country as a global manufacturing superpower (White House, 2025), a significant threat looms in the form of industrial knowledge loss.
The industrial sector is facing a severe labor crisis. As the workforce ages and retires, decades of accumulated expertise are disappearing, creating a widening knowledge gap. According to the Bureau of Labor Statistics, 31% of service engineers are over the age of 55. Mckinsey reports that 43% of manufacturing job openings remain unfilled. According to the Bureau of Labor Statistics, 31% of service engineers are over the age of 55.43% of manufacturing job openings remain unfilled. According to the Bureau of Labor Statistics, 31% of service engineers are over the age of 55.
This loss of institutional knowledge leads to:
- Lost revenues – As 23% of machine downtime is caused by human errors, the staggering loss of U.S manufactures estimates at $92B.
- Lost business opportunities – Companies often hesitate to expand or sell new equipment due to concerns about inadequate service resources, fearing they won’t meet their target SLAs, which limits revenue growth and market competitiveness.
- Operational inefficiencies – Less experienced workers struggle to diagnose and fix complex machinery issues, increasing downtime. A Siemens report reveal that recovery time from downtime has increased by 60% over the past five years.
- Increased safety risks – A lack of experience-based, tribal knowledge of best practices leads to higher accident rates. According to a Deloitte report, predictive maintenance and workforce training gaps significantly contribute to operational safety concerns in industrial settings.
Additionally, manufacturers are struggling to fill vacancies. The National Association of Manufacturers projects that 2.1 million industrial jobs will remain unfilled by 2030, further exacerbating the knowledge gap. Without proactive measures, companies will face higher costs, lower productivity, and a continued decline in operational efficiency.
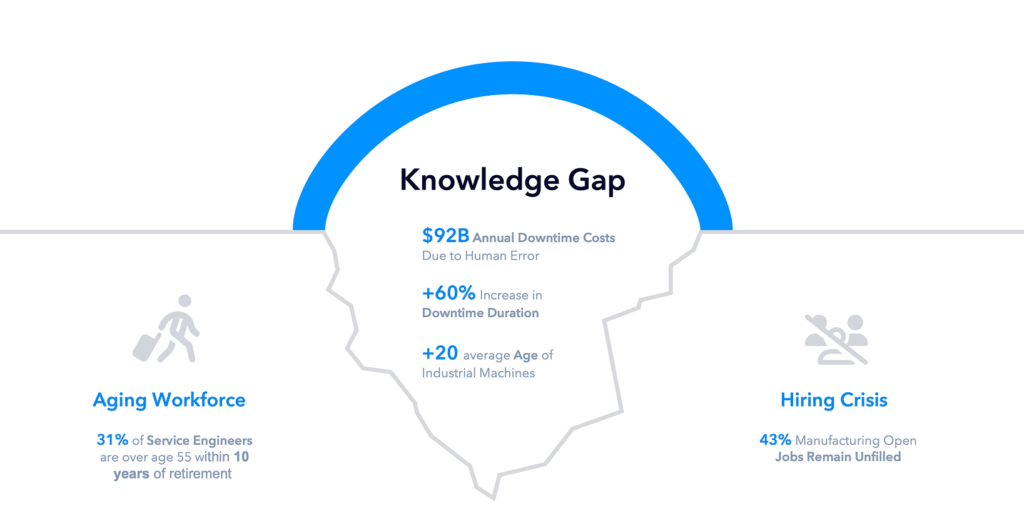
Part 2: Why Traditional Enterprise AI Tools Fail to Solve This Problem
Many industrial companies turn to AI for solutions, but generic AI platforms often fail to address sector-specific challenges. Traditional enterprise AI struggles because:
- Inability to capture “tribal” industrial knowledge: Industrial expertise is often buried in decades-old manuals, maintenance logs, and service records. Additionally, much of this knowledge remains undocumented, existing only in the minds of experienced workers who are nearing retirement. Without the ability to capture and structure this expertise, AI-driven automation remains unreliable. For example, an experienced technician might have developed a workaround for a recurring machine fault that isn’t documented in service manuals. If this knowledge isn’t captured and institutionalized, newer employees will lack the insights needed to resolve critical issues efficiently, leading to prolonged downtime and increased costs.
- Limited Accuracy and Poor Source Transparency: In industrial environments, even a minor misinterpretation of a process or equipment instruction can lead to costly downtime, safety hazards, and compliance violations. Many enterprise AI models lack the precision and contextual awareness required for high-stakes decision-making, making them unreliable for mission-critical operations.
Most AI knowledge management platforms lack transparency and contextual depth. They often fail to cite sources, making it difficult for users to validate AI-generated insights. This undermines trust and limits the system’s reliability. More critically, these platforms typically restrict access to the original materials—such as technical diagrams, tables, or training videos—where essential operational details reside. Without this full context, frontline teams are left without the actionable information they need to perform their work safely, accurately, and efficiently.
- Security and infrastructure concerns. Industrial organizations operate under strict compliance requirements, and many facilities lack the IT infrastructure to support fully cloud-based AI systems. As a result, companies require flexible deployment options, including on-premise or hybrid solutions, to maintain security while benefiting from AI advancements.
Traditional enterprise AI tools are not built to effectively manage industrial knowledge. They struggle to capture and retain both structured and undocumented expertise, lack the zero-error tolerance required for manufacturing environments, and often fail to meet strict security and compliance standards. For example, a generic AI system used in an industrial setting may misinterpret machine log data or fail to recognize critical troubleshooting steps known only to veteran technicians, leading to costly errors and inefficiencies. This highlights the urgent need for AI solutions that are purpose-built for industrial applications, ensuring accuracy, security, and knowledge preservation.
Part 3: What an Effective Knowledge Management Platform Should Look Like
An effective knowledge management platform for industrial organizations must do more than just store information—it must actively capture, structure, and provide seamless access to critical knowledge. By integrating AI-driven organization, continuous learning, multi-modal access, and enterprise-grade security, companies can preserve expertise, enhance operational efficiency, and reduce costly downtime. The following components are essential for a robust knowledge management solution:
1. Industrial Knowledge Orchestration
A successful industrial knowledge system must capture and organize different types of knowledge, ensuring accessibility and usability across an organization. This includes:
- Structured content in legacy formats – Documents, presentations, videos, and spreadsheets that contain critical operational and procedural knowledge.
- Unstructured documented content – Service case notes, machine logs, and other informal records that capture troubleshooting insights and real-time operational data.
- Undocumented tribal knowledge – Expertise residing in the minds of subject matter experts (SMEs) that is often not formally recorded but is essential for maintaining operational efficiency and best practices.
Rather than solely processing historical data, AI should learn from ongoing interactions within an industrial environment. As employees engage with AI-driven knowledge systems, their inputs, feedback, and problem-solving approaches should be captured, refined, and reintegrated to enhance system accuracy and relevance.
For example, an AI system deployed in a manufacturing plant could analyze equipment performance data, frontline worker feedback, and past service logs to improve root-cause-analysis insights. If a technician discovers a more efficient way to address an issue, the AI should capture that insight, validate its effectiveness, and incorporate it into future recommendations. Over time, this iterative learning process ensures that AI remains aligned with real-world operational nuances, making it an invaluable tool for knowledge retention and process optimization. By leveraging AI, organizations can extract, structure, and preserve this knowledge, ensuring seamless transfer and utilization across their workforce.
2. Specialized Industrial AI Models for Multi-Modal Execution
To deliver operational value, AI systems must be more than general-purpose engines—they need to be trained and tuned specifically for the complex nature of industrial knowledge. This includes building models that are fine-tuned on a company’s proprietary data, workflows, and documentation.
These models must be capable of understanding and responding to multi-modal inputs such as technical documents, diagrams, videos, and logs. Unlike off-the-shelf tools, these specialized models provide contextualized answers grounded in the real-world complexity of operations, engineering, maintenance, and safety.
Furthermore, a robust knowledge management platform must support a multi-modal user experience, allowing users to access sources in their original format and exact location. This ensures credibility, enables validation, and provides the critical context necessary for execution. By integrating these specialized, multi-modal AI models, organizations can bridge the gap between high-level guidance and actionable, validated insight on the frontlines.
For example, an AI-powered system should be able to open a document while preserving its original visuals, diagrams, and tables, ensuring engineers can accurately interpret technical specifications. Similarly, a training video should be accessible within the platform, allowing users to see precise procedural steps for complex tasks. By integrating this multi-modal capability, industrial teams can verify AI-generated insights, improve accuracy, and execute tasks with confidence.
3. Industrial-Grade Security and Compliance
Some manufacturing firms operate under strict regulatory frameworks, but for all, meeting security and compliance standards is a fundamental requirement. AI solutions must adhere to data privacy laws and industry regulations, including:
- ISO 27001 – Information security management standards.
- SOC 2 – Framework for managing customer data based on security, availability, processing integrity, confidentiality, and privacy.
- GDPR & CCPA – Regulations ensuring compliance with global and regional data privacy laws.
Additionally, many industrial organizations have concerns about cloud-based solutions due to stringent security requirements. As a result, many prefer on-premise or hybrid AI solutions that provide greater control over data security and compliance.
Summary
In summary, an effective industrial knowledge management platform must unify four critical pillars: comprehensive knowledge orchestration, adaptive AI learning loops, multimodal content access, and robust enterprise-grade security. Together, these elements empower organizations to preserve hard-won expertise, drive operational precision, and deliver consistent execution at scale.
Closing Thoughts
As the U.S. manufacturing sector seeks to reclaim its position as a global industrial leader, knowledge loss from an aging and shrinking workforce presents a hidden but urgent challenge. Generic AI tools fall short in addressing the complexity and specificity of industrial environments. Purpose-built platforms that capture and activate institutional knowledge across media, users, and workflows will define the next generation of high-performing, resilient manufacturers. Those who act now will gain a lasting competitive advantage in the era of intelligent industry.